[vc_row][vc_column][vc_column_text]
I think we need a new way to talk about artificial intelligence in the law.
I’m seeing a lot of frustration and cross-talk lately in the legal innovation community around what does and doesn’t constitute legal AI, and whether it will or won’t deliver any real value.
The term “legal AI” isn’t helping much: it’s vague and wooly enough to mean almost anything, and its sci-fi connotations raise expectations beyond what any technology can realistically deliver at this point. I think we need to go back to basics and deconstruct what we’re trying to achieve with this technology, and why.
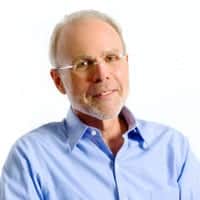
While I was thinking about this subject, the May 2018 edition of the ABA’s Law Practice Today arrived in my inbox, featuring a remarkably on-point article by Michael Mills of Neota Logic. Michael answers the question “What is AI?” with this retort: “First, AI isn’t really ‘artificial’—it’s all created by humans through very, very hard work — and it isn’t really ‘intelligent’ either — the software doesn’t know what it’s doing or why.
“Second, AI is not a ‘what.’ We can’t point to anything and say, ‘Yup, that’s an AI, right over there by the door.’” (Advance thanks to Michael, who’s provided thoughts on this post in draft form.)
So, what are we really talking about when we talk about legal AI? “A large and growing collection of mathematical methods embodied in software for doing narrowly defined but very useful [legal] tasks,” is Michael’s apt description. This suggests to me that we ought to look more closely at the tasks in question, in order to find out why we’re applying these methods to accomplish them.
Michael classifies these tasks into five categories:
- Electronic Discovery
- Legal Research
- Analytics & Prediction
- Expertise Automation
- Contract Analysis
Why use “legal AI” to carry out these tasks? Michael suggests that these applications of AI can enable lawyers to:
- Serve more clients more effectively at lower cost.
- Create new revenue streams not dependent on hours worked.
- Focus time and expertise on work that requires the uniquely human and professional skills of empathy, judgment, creativity, and advocacy.
- Increase access to justice by meeting the legal needs of the poor and middle class.
Client Benefits
This all seems accurate to me. I’d like to take the inquiry a step farther and ask: What are the benefits to clients of applying these methods to these tasks?
I wrote last year that lawyers should evaluate any potential AI investment in terms of whether and to what extent clients will benefit. In that spirit, I’d like to suggest a client-centred framework for viewing legal AI.
It seems to me that the five sets of tasks Michael has enumerated can themselves be broken down into two general categories.
1. Volume and Costs
One category contains all those tasks that “legal AI” can accomplish in less time and at lower cost than human lawyers can.
Put differently, these are the tasks that human lawyers could carry out — indeed, in the not-too-distant past, routinely carried out — but that would exact an enormous cost if they were left to humans today. You might think of these as “volume” tasks: If you put a million lawyers to work on these tasks, and gave them plenty of time, they could do a fine job.
- Electronic discovery is the ideal example here. Theoretically, sure, lawyers alone could identify all the relevant documents hidden in terabytes of e-data, and the results they achieved likely would not differ substantially from what e-discovery software could accomplish. But of course, the costs of this approach are mind-boggling: no judge would authorize it and no client would pay for it if there were any other way to carry it out.
- Or take contract analysis. Multinational A buys Multinational B, and the resulting Himalayan pile of contracts needs to be identified, reviewed, analyzed, and rationalized for various purposes. Give me a million lawyers with a million hours each, and I’ll render just as good a result as a cognitive-reasoning software program — so long as the merger can wait 40 years and the value of the new corporate behemoth can somehow afford the lawyers’ fees.
- For the most part, I think you could also add legal research to this category. A million lawyers, each armed with a million-hour quota, could review every case in existence and gradually work their way down to identify the most salient decisions for a judge’s consideration. Mathematical-model methods can do the job at a sliver of a fraction of the cost, and while that’s not the entirety of legal research by any means, this aspect of it fits the bill here.
These three types of legal tasks are susceptible to the application of legal AI, not because the AI produces better results, necessarily — though if you want to argue that machines are less error-prone and inconsistent than overworked lawyers with aching eyes and mental fatigue, go right ahead — but because it produces substantially similar results at an enormously lower cost of effort, money and time.
That’s what matters to clients. So the client-centred rationale for using these methods is “Reduce costs.”
2. Expertise and Scarcity
But then there’s the second category of tasks, and this one is much more interesting. This category contains all those tasks that “legal AI” can accomplish by imitating or replicating lawyers’ logical, analytical, and advisory skills. If the first category of tasks was about “volume,” this one is about “expertise,” and the value propositions here are very different.
Take the group of tasks that Michael refers to as “expertise automation.” This is a fascinating area in which Michael’s own company, Neota Logic, has been a pioneer: he describes it as “the automation of substantive legal guidance and processes … [that] combines expert systems and other artificial intelligence techniques, including on-demand machine learning, to deliver answers to legal, compliance, and policy questions.”
Note that the tasks given to expert systems are not “volume tasks.” You could assign a million lawyers to answer a client’s question, but you’re not necessarily going to get the right answer, because maybe none of these lawyers has the expertise required to give the right answer.
What you need is an expert lawyer who knows this area of law and will ask clients the right questions, tap into the appropriate set of facts and experiences, follow the correct reasoning path, and render an accurate response.
Here’s the client’s problem: this kind of expertise is scarce. Only a relative handful of lawyers possess the knowledge, experience, and skill to answer specific kinds of client questions, and the narrower the field of expertise, the smaller the number. The scarcity of this resource raises its price, restricts its accessibility, and renders it prone to charging by the hour.
But suppose the client could distill this expertise into a complex database of probabilistic reasoning and computational decision trees that could provide substantially the same answers that the lawyer would give.
This program would be invaluable to the client because it would increase the supply — and reduce the scarcity — of legal expertise.
This is, in fact, exactly the situation that Profs. Richard and Daniel Susskind describe in their recent book The Future of the Professions.
In the Susskinds’ view, the historical influence and dominance of the professions is substantially grounded in the exclusivity that professionals maintain over access to expert knowledge and the ability to dispense it.
Richard has stated that the book really seeks to answer the question: “How do we produce and distribute practical expertise as a society?” This is not a new topic: as far back as 2000, he defined an expert system as “the use of computer technology to make scarce … expertise and knowledge more widely available and more easily accessible.”
We might even be standing on that threshold today. Here’s what Ben Hancock of The Recorder reported from the LegalWeek 2018 legal technology conference earlier this year:
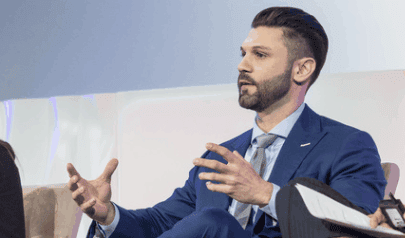
Brian Kuhn, the global leader and co-founder of IBM Watson Legal, envisions — and it sounds like IBM is implementing — the creation of “cartridges” of specialized legal information that can be deployed for various legal tasks. That’s a mouthful, I know.
But imagine this: A firm that specializes in antitrust law “trains” an AI algorithm to interpret documents relevant to that practice area. Then, the firm sells that piece of trained software, allowing a firm weak in antitrust to gain capacity (and removing the need, perhaps, to bring on a bunch of antitrust partners).
Now IBM, it’s true, perpetually seems to be “a few years away” from releasing a game-changing legal technology breakthrough. But an “expertise cartridge” is exactly what we’re talking about here: distilled legal know-how, transferrable from user to user, distributed widely — the “democratization” of legal expertise, if you want to get political about it. And the primary buyer for a product like that wouldn’t be “a law firm weak in antitrust,” but the GC of a large corporation, who would be very interested in a 24/7, mobile, and scalable source of antitrust expertise.
The same analysis would apply to our fifth category of legal AI tasks, “analytics and prediction.” I’m being persuaded to the view, recently enunciated by Sarah Sutherland and Sam Witherspoon among others, that we’re not going to achieve effective litigation prediction from the distillation of court decisions alone — the data points are too few and insufficiently robust.
But in broad terms, “outcome prediction” is really the archetypal, fundamental lawyer functionality: To answer the recursive client question, “What’s going to happen in my situation?” Again, from the client perspective, this isn’t a problem of volume, but of the scarcity of resources available to answer a legal question.
Reality Check
Now, let’s pull back for a moment and write ourselves a reality check. We don’t have the means today to build programs that can render detailed legal analyses of complex problems or advise with statistical confidence where clients’ current legal circumstances are likely to lead them. Nor are we remotely on track to get there.
But if you want to know what the Holy Grail of Automated Legal Services looks like, that’s it. And considering the potential payoff for anyone who finds it, you know that a lot of smart people are trying to find the right combination of jurisprudential data, trial lawyer experience, arbitration outcomes, negotiation principles, tribunal decisions, and human game theory that will unlock this prize.
A New Framework
So, let’s return to the goal I set for myself at the start of this post: Figuring out a better framework for talking about AI in legal services. I would suggest we classify legal AI offerings according to the type of market problem they aim to solve. I’ve proposed two categories of these problems:
- The volume costs of lawyer effort
- The scarcity costs of lawyer expertise
There are probably others, or you could break these categories down into finer classifications, but it should do for a start. Here’s a basic matrix of these problems, their proposed AI solutions, and the likely impact of those solutions on lawyers and law firms.
Finally, because everyone loves fighting over nomenclature, here’s a potential naming protocol.
- I’d suggest the term “Volume AI” for those applications that accomplish high-volume legal tasks far more quickly and efficiently than human lawyers do, to generate great cost and time savings for clients.
- I’d suggest the term “Expertise AI” for those applications that make scarce legal expertise widely available in computerized form, to generate greater accessibility for clients to the legal answers they need.
“Volume AI” would refer to any technology that reduces the time and effort of lawyers; “Expertise AI” would refer to any technology that increases the accessibility to clients of valuable but scarce legal expertise. (Maybe we can eventually do away with “AI” altogether in this area, but let’s start small.)
That’s my proposed framework for thinking and talking a little differently about legal AI. The comments section is open for your thoughts.
Author –
*Jordan Furlong is a leading analyst of the global legal market and forecaster of its future development. Law firms and legal organizations consult him to better understand why the legal services environment is undergoing radical change and to advise their lawyers how to build sustainable and competitive legal enterprises that can dominate the new market for legal services.
[/vc_column_text][/vc_column][/vc_row]